One thing I’ve learned from moonlighting as a Dasher — and I’ve started implementing at Oatfin — is that you have to measure everything. You can’t just go by gut feeling.
For example, DoorDash tracks everything: delivery times, restaurant wait times, driver acceptance rates, how long to wait for an order, even how weather affects order volume. They also measure how effective their customer support is by sending a survey after each interaction.
As a Dasher, you naturally start thinking the same way:
– Which zones are most profitable?
– What days and times maximize order flow?
– Which orders are worth it?
– What’s the fastest route?
– How long does each stop really take?
– Which neighborhoods tend to tip better?
– Which restaurants to avoid?
For example, in the beginning, I used to reject offers based on instinct. Now, I quickly plug in the address on Google Maps and know exactly how long it’ll take. Data wins.
That data-driven mindset is exactly what we bring to Oatfin.
We measure the things that matter to developers — the signals that reveal real friction and real progress:
– How long does each deployment take?
Every minute shaved off a deployment means faster iteration, tighter feedback loops, and happier developers. We track deployment duration across environments so teams know exactly where their bottlenecks are.
– How much money is saved?
Automation isn’t just about speed — it’s about efficiency. We monitor the actual cost savings from optimizing workflows, reducing manual toil, and minimizing cloud waste. Developers can see the tangible ROI of the systems they build.
– How long does it take to resolve an incident?
Time-to-resolution is everything during a fire. We capture how long it takes from alert to resolution, what slowed things down, and how similar incidents can be prevented in the future.
– Where users get stuck, and why
Whether it’s onboarding a new service, setting up automation, or debugging an issue — we identify the exact friction points in the developer journey. Then we fix them. Fast.
Because great developer experiences don’t happen by accident — they happen by design. And design starts with data.
Every data point is a chance to improve. And when you’re building for speed, scale, and reliability—guesswork isn’t an option.
Author: Jay Paulynice (Page 1 of 3)
The reality of working on a startup is that sometimes things happen at a glacial pace.
One day, you’re making rapid progress—closing a deal, shipping a big feature, landing a key partnership. The next, you’re waiting. A contract drags on. A customer takes weeks to decide. An investor conversation that seemed promising goes quiet.
It’s a weird contrast. Startups are often talked about in terms of speed, but a lot of the work is just patience—showing up every day, even when nothing seems to be moving.
I share this because there is actually a lot of bad advice out there. For example, some investors say you have to be growing X percent weekly or monthly. When I first started my startup journey, I thought investors knew better and when I didn’t see progress, I stopped working on my ideas.
With Oatfin, I decided to take a different approach. I focused on consistency over arbitrary growth targets. Instead of obsessing over weekly percentage gains, I committed to showing up every day, solving real problems, and delivering value to customers.
Some weeks, growth is explosive. Other weeks, it’s just steady progress—improving the product, strengthening relationships, or laying the groundwork for future wins. And that’s okay.
The truth is, startups aren’t built in sprints; they’re built in sustained, deliberate effort over time. Patience, persistence, and adaptability matter just as much as speed.
2024 was a mini breakthrough year for Oatfin content marketing wise. We made significant strides in growing our presence organically on LinkedIn.
Here’s what we achieved:
✅ 114,387 post impressions
✅ 40,562 LinkedIn members reached
✅ 1,013 page followers
✅ 131 newsletter subscribers
But we’re just getting started.
My vision for 2025 is ambitious, and I’m excited to take it to the next level:
🎯 1M+ post impressions
🎯 100,000+ LinkedIn members reached
🎯 2,000 page followers
🎯 500 newsletter subscribers
Thank you for being part of this journey! Your engagement, feedback, and support drive us to create even more impactful content for this incredible community.
Here’s to an even bigger and better 2025!
-Jay
Thank you for subscribing to Cloud Musings! If you haven’t subscribed yet, we’d love to have you join us to stay updated on our journey and insights into cloud innovation.
As 2024 comes to a close, we’re thrilled to look back on a year of incredible growth, innovation, and milestones for Oatfin. This year has been pivotal in solidifying our position in the cloud infrastructure space, and we couldn’t have done it without our amazing community.
Highlights of 2024:
- Scaling Innovation: We introduced Oatfin Observability, which leverages cutting-edge generative AI to streamline observability data for rapid incident resolution. Alongside this, our core platform saw significant enhancements based on user feedback, making Oatfin even more indispensable for cloud teams.
- New Horizons in Cost Efficiency: We launched Oatfin Cloud Cost Intelligence, enabling customers to unlock collective savings on cloud expenses—yet another step toward making cloud infrastructure more accessible and cost-efficient for everyone.
- Impressive Growth: Oatfin achieved 2.5x revenue growth this year, reaching $616K ARR, and expanded our customer base significantly, validating our mission to simplify cloud application delivery.
- Content Marketing: Our content marketing has reached 40,575 LinkedIn members with 114,501 views, driving awareness and engagement. In addition, our LinkedIn page reached 1,013 followers. Our focus on thought leadership and actionable insights has built trust and showcased our innovation to cloud, DevOps professionals, and the greater startup ecosystem.
- Customer Success Stories: We’re humbled by the stories from customers who’ve used Oatfin to save time, reduce costs, and enhance productivity. Your success is what drives us every day.
- Fundraising Success: We closed our first angel round and are well on our way to completing our $3M seed round, setting the stage for the next chapter of growth and innovation.
Navigating Challenges:
This year, we faced an uncertain economic landscape, but we remained steadfast in our goals. By focusing on operational efficiency, customer value, and staying true to our mission, we’ve not only weathered the challenges but have come out stronger, more focused, and ready for the future.
Looking Ahead to 2025:
- Accelerating Product Innovation: With fresh funding, we’re doubling down on building transformative generative AI solutions for cloud infrastructure, giving enterprises tools that deliver insights and results faster than ever before.
- Market Leadership: Our focus on partnerships and strategic initiatives will help us expand our footprint across industries and regions, empowering more businesses to embrace Oatfin.
- Commitment to Sustainability and Community: We remain deeply committed to fostering responsibility in tech, giving back to our community, and helping customers achieve their goals sustainably.
Thank You:
As we close out 2024, we want to express our deepest gratitude to our customers, investors, and partners. Your support has been the foundation of everything we’ve achieved this year, and we’re more motivated than ever to make 2025 even more impactful.
Wishing you a joyful holiday season and a prosperous New Year!
Warm regards,
-Jay
Reflecting on life, I wish I had failed more in my early years.
I know what you’re thinking: Who wishes for failure?
But hear me out.
In my early years, I just “got” things. I was a straight-A student in high school. College wasn’t exactly a breeze, but it also wasn’t particularly difficult. Grad school followed a similar pattern.
Here is a concrete example: Most multiple choice questions have 5 choices, which means the average test taker has a 20% chance of picking the right answer. But if you notice closely, there is almost always 2 answers that are extremely close and so by figuring this out, you increased your chances of picking the right answer to 50%.
Looking back now, I realize I wasn’t really challenged.
Sure, I worked hard, but I rarely found myself in situations where I had to pick myself up after failing or truly wrestle with something I couldn’t immediately grasp. It was only later, in the real world—where stakes are high, ambiguity reigns, and “failure” is part of the job description—that I learned just how valuable those early struggles could have been.
Failure, I’ve come to understand, is a gift. It’s humbling, but it’s also where the growth happens. It teaches resilience, creativity, and grit—qualities you can’t develop when things always come easy.
The irony is that I’ve achieved some of my greatest successes because of my failures later in life. Whether it’s been building a company, navigating relationships, or simply becoming a better version of myself, those failures forced me to adapt, learn, and grow in ways that my early “successes” never did.
If I could go back in time, I would attend college in my senior year of high school because I was taking all AP classes at that point.
I’d also tell my younger self to take more risks, step out of my comfort zone, and welcome the possibility of falling short. Because that’s where the real lessons live.
As the year draws to a close, I find myself once again reflecting on the remarkable journey of building Oatfin. Each year brings its own set of challenges and triumphs, and through it all, gratitude remains at the heart of this experience.
Here’s what I’m deeply thankful for as we continue to grow:
1. Unwavering Support
To the mentors, advisors, friends, and family who’ve been there from the beginning, thank you for being our steady anchors. Your guidance, encouragement, and unwavering belief in us have been nothing short of life-changing.
2. Our Amazing Customers
To our early adopters and loyal customers, you are the foundation of everything we do. Your feedback, trust, and engagement inspire us to innovate and deliver beyond expectations. We are building this with and for you, and your support means the world.
3. Personal Growth
Entrepreneurship continues to be the most intense personal growth journey. I’m grateful for the lessons—both exhilarating and humbling—that have shaped me as a leader, a founder, and a person.
4. Community and Ecosystem
The startup ecosystem is like no other. From investors who took a chance on us to the peers who’ve shared insights and experiences, I’m thankful for the collective wisdom and camaraderie that make this path so rewarding.
5. Validation and Momentum
Seeing our vision resonate with the market and gaining traction this year has been a thrilling reminder that the hard work is paying off. It’s a privilege to be building something that solves real problems and adds value.
6. The Oatfin Team
This year, I’m especially grateful for the incredible team we’ve built. Your dedication, creativity, and resilience have been the driving force behind every success. Thank you for believing in the vision and pouring your heart into making it a reality.
7. Family
Lastly, to my family—thank you for your patience, love, and sacrifices. To my sons, who inspire me every day with their curiosity and dreams (building PCs and aiming for Harvard!), you remind me why I do this and who I do it for.
As we prepare to close another chapter and step into a new year, I’m filled with optimism for what lies ahead. Here’s to continued growth, new challenges, and the unwavering gratitude that keeps us grounded.
Thank you all for being part of this incredible journey.
— Jay
They say life begins at 40, but what does that really mean?
For many, this milestone prompts a mix of emotions—nostalgia for the past, curiosity about the future, and a sense of urgency about the present. As I’ve navigated this chapter, I’ve come to realize that life after 40 isn’t about slowing down; it’s about evolving. It’s about embracing growth, living with intention, and finding fulfillment in ways that once seemed elusive.
Gaining Clarity
One of the biggest surprises of turning 40 was the clarity it brought. In my 20s, I was still figuring out what I wanted, and in my 30s, I was building relentlessly—career, family, dreams. But now, there’s a sharper focus. I know what truly matters to me, and I’ve stopped chasing things that don’t align with those priorities. It’s liberating to set aside distractions and pour my energy into what counts.
“If the 20s are for exploration and the 30s are for building, the 40s are for refining.”
Embracing Confidence
In the past, I cared too much about how I was perceived—whether I was doing “enough” or succeeding in the “right” way. But something shifts after 40. There’s this quiet confidence that comes from knowing who I am and what I’m capable of. I’ve learned to embrace my strengths, acknowledge my flaws, and pursue things that light me up—without needing validation from anyone else.
Learning to Value Health
I won’t lie—there’s a stark difference in how my body feels now compared to my 20s or even my 30s. The midnight snacks or skipped workouts don’t bounce back the same way. But instead of resenting it, I’ve learned to value my health in a deeper way. Exercise and good nutrition have become more than just habits—they’re investments in the energy and longevity I need to enjoy life fully.
Deepening Relationships
One of the greatest gifts of this stage in life is the relationships that have endured. I’m more selective about where I invest my time and emotional energy, focusing on the connections that bring joy, depth, and meaning. Whether it’s being more present for my kids, strengthening my marriage, or catching up with lifelong friends, these relationships remind me what life is really about.
Redefining Success
In my 20s, success was about climbing the ladder. In my 30s, it was about building something sustainable. Now, success feels more personal. It’s about impact—what I’m creating and contributing to the world. It’s about making sure the work I do aligns with my values and leaves a lasting legacy. It’s a shift from “How much can I achieve?” to “How meaningful can my contributions be?”
The Prime of Life
Life after 40 isn’t about a midlife crisis; it’s about a midlife awakening. There’s still so much to learn, explore, and enjoy, and I’m grateful for every opportunity to keep growing. This is a chapter where wisdom meets possibility, and that’s an incredibly exciting place to be.
At Oatfin, we’ve been hard at work crafting a robust architecture that powers our web applications to handle millions of concurrent users and deployments seamlessly. In this blog, we’re thrilled to unveil the architecture behind the scenes, showcasing the technologies and strategies that drive our platform’s success.
Architecture Overview:
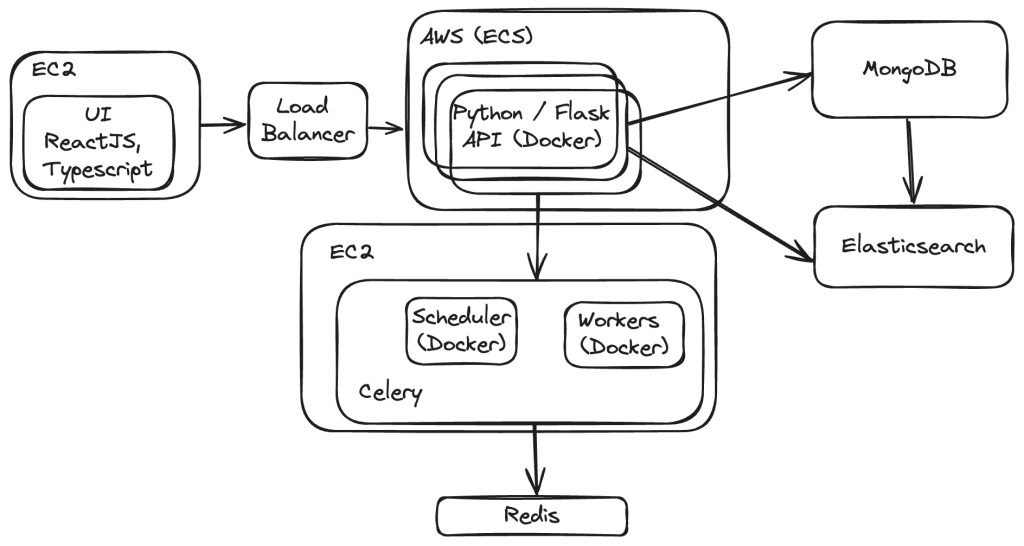
Let’s dive into the heart of our architecture. At Oatfin, we’ve crafted a solid foundation comprising key components:
- React, Typescript UI deployed on an EC2 instance: Our user interface is built using React and Typescript, providing a seamless and dynamic user experience. Deployed on Amazon EC2 instances, our UI delivers high performance and responsiveness to users worldwide.
- Python, Flask API using Docker and deployed using AWS Elastic Container Service (ECS): Powering our backend, we rely on Python’s robustness and the elegance of Flask to develop our APIs. Leveraging Docker containers, we ensure consistency and scalability in our deployments. AWS Elastic Container Service (ECS) manages our containerized applications, offering effortless scalability and reliability.
- MongoDB database using MongoDB Atlas: Data is the lifeline of any application, and at Oatfin, we entrust MongoDB to store and manage our data efficiently. With MongoDB Atlas, we enjoy the benefits of a fully managed database service, including automated backups, security features, and seamless scalability.
- Elasticsearch for indexing and searching documents: Searching and indexing documents is a breeze with Elasticsearch. We utilize its powerful search capabilities to enhance the discoverability of content within our applications, delivering fast and relevant results to our users.
- Celery, Redis task queue, and scheduler running inside Docker and deployed on an EC2 instance: Task queuing and scheduling are essential for handling background processes and asynchronous tasks. With Celery and Redis, we orchestrate our tasks efficiently, ensuring optimal performance and resource utilization. Docker containers simplify the deployment and management of Celery and Redis components, while EC2 instances offer the flexibility and scalability we need.
Scalability and Deployment:
One of the hallmarks of our architecture is its scalability. By leveraging cloud-native technologies and best practices, we’ve built a platform that can effortlessly scale to meet growing demands. Whether it’s handling spikes in user traffic or deploying updates seamlessly, our architecture is designed to adapt and grow with ease.
Our deployment process is streamlined and efficient. We deploy our React UI on EC2 instances, containerize our Flask API using Docker, and leverage AWS ECS for orchestration and scaling. MongoDB Atlas and Elasticsearch further simplify our deployment, offering managed services that eliminate infrastructure overhead.
Challenges and Future Improvements: Of course, building and scaling a complex architecture like ours comes with its share of challenges. From optimizing performance to ensuring security and reliability, we continuously strive to overcome obstacles and improve our platform.
Looking ahead, we’re excited about the future possibilities. We’re exploring new technologies, optimizing our architecture for even greater performance, and refining our processes to deliver an exceptional user experience.
At Oatfin, we’re proud of the architecture we’ve built, and we’re excited to share it with you. Our commitment to scalability, reliability, and innovation drives us forward as we continue to push the boundaries of what’s possible. Thank you for joining us on this journey, and we look forward to shaping the future of technology together.
It’s been an exciting 6 months here at Oatfin. Since we launched Oatfin Cloud Cost Intelligence in beta, we’ve received invaluable feedback and I wanted to share our progress.
Feedback that Drives Innovation:
First and foremost, a huge thank you to everyone who jumped into the beta testing phase with us. The feedback has been invaluable! We’ve been diligently listening, learning, and refining Oatfin Cloud Cost Intelligence based on your insights. Your input is shaping the future of our platform, and we couldn’t be more grateful.
What We’ve Learned:
Our beta users have highlighted specific pain points and challenges they face in managing AWS costs. Unpredictable costs, hidden fees, and the lack of visibility are consistent themes. Your experiences are the driving force behind our mission to simplify and optimize cloud cost management.
Refining the Intelligence:
Since the beta launch, we’ve been hard at work fine-tuning the AI models, enhancing data collection processes, and optimizing our approach to better predict and manage cloud costs. The journey to solving the cloud cost puzzle is ongoing, and the feedback is the compass guiding us in the right direction.
Exciting Features on the Horizon:
Based on your suggestions and needs, we’re gearing up to roll out some exciting features in the coming weeks. Stay tuned for updates on enhanced cost visibility, personalized recommendations, and even more ways to take control of your AWS spending.
How You Can Help:
Your continued participation in the beta testing phase is crucial. Please keep those insights coming! Whether it’s a small observation or a big “Aha!” moment, every bit helps us refine and improve Oatfin Cloud Cost Intelligence.
Join the Conversation:
We’ve set up a dedicated space for beta users to share thoughts, ask questions, and connect with each other on Slack. Your experiences and expertise contribute to a vibrant and collaborative Oatfin community.
Thank you for being part of this journey with us. Together, we’re reshaping the way cloud costs are managed.
Stay tuned for more updates, and as always, feel free to reach out if you have any questions or thoughts to share.
Best,
Jay
Thank you for subscribing to Cloud Musings! If you haven’t subscribed yet, please consider subscribing to receive automatic updates when I publish new editions.
As we approach the close of another transformative year, we are delighted to reflect on Oatfin’s remarkable journey in 2023. It’s been a year filled with growth, challenges, and unwavering commitment to our mission.
Highlights of 2023:
- Product Evolution: Based on invaluable feedback we received from our users, we launched Oatfin Cloud Cost Intelligence in beta, which is designed to simplify cloud cost management on AWS. In addition, we made a lot of improvements and added a lot of new features to our Oatfin Cloud product.
- Cloud Musings Newsletter: In 2023, we launched the Cloud Musings newsletter on LinkedIn and Substack. We’ve seen a number of subscribers and growth in the Oatfin community.
- Financial Milestones: We’re proud to report a 2.3x growth in revenue this year, underscoring Oatfin’s resilience and market relevance. Our continued focus on cloud infrastructure has yielded promising results, setting the stage for sustainable growth.
- Customer Success: We’ve received positive feedback from our valued customers, reaffirming the impact and value of our solutions in their operations.
Navigating Challenges and Embracing Opportunities:
While the year presented its set of challenges from the fundraising environment, our dedication and adaptability ensured that we turned obstacles into opportunities. Through strategic planning and collaborative efforts, we’ve overcome hurdles, gaining invaluable insights and experience along the way.
Looking Ahead: A Glimpse into 2024:
- Fundraising: We’re on track to conclude our seed fundraising, paving the way for accelerated growth and innovation.
- Innovation: We’re gearing up to expand our product suite, integrating cutting-edge generative AI solutions.
- Market Expansion: Through strategic partnerships and initiatives, we aim to fortify our market footprint.
- Excellence in R&D: Our commitment to innovation remains unwavering, with increased investments in research & development and customer experience.
- Sustainability & Community: Rooted in our core values, we’re committed to fostering sustainability, responsibility, and active community engagement.
In Conclusion:
As we conclude 2023, we extend our heartfelt gratitude to our loyal customers, partners, and stakeholders. Your unwavering support, collaboration, and trust have been instrumental in Oatfin’s success, and we look forward to achieving new milestones together in the coming year.
Wishing you a joyful holiday season and a prosperous New Year!
Warm regards,
Jay